Accelerating AI Adoption with Ramsay Brown
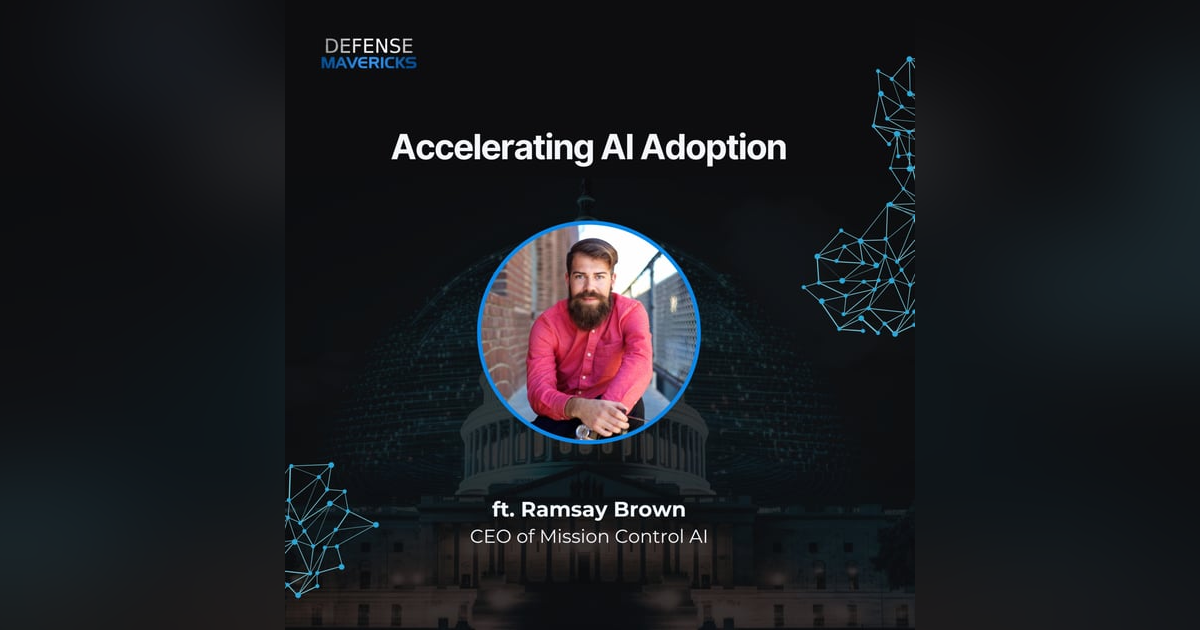
This week, Bonnie sits down with Ramsay Brown, CEO of Mission Control AI, at DoD Advantage 2024 to talk about how to keep up with the rapid advancements in AI. Ramsay dives into the impact of AI on industries and personal lives, the looming need for responsible AI governance, and the challenges that organizations face when adopting these technologies. He also shares concrete strategies for maintaining human-machine hybrid effectiveness and provides his insights into how we can accelerate the adoption of AI. Tune in for a fun conversation on the future of AI integration.
TIMESTAMPS:
(2:56) Figuring out the “so what” of tech
(4:16) Finding the intersection of new tech and daily life
(7:44) Superficial trust vs. deep trust in LLMs
(11:14) Using continuous verification for ethical alignment
(15:50) Why LLMs fail
(17:09) Why AI impacts all digital work, despite low adoption rate
(21:39) How AI models will rapidly advance the future of work
(26:23) Creating an AI-built future
(29:23) Distinguishing the “smell” of generative AI
(31:09) How responsible AI leads to tech alignment
(35:24) Building a network of friends
LINKS:
Follow Ramsay: https://www.linkedin.com/in/ramsaybr/
Follow Bonnie: https://www.linkedin.com/in/bonnie-evangelista-520747231/
CDAO: https://www.ai.mil/
Tradewinds AI: https://www.tradewindai.com/
Mission Control AI: https://usemissioncontrol.com/
Bonnie Evangelista [00:00:02]:
All right, welcome. This is Bonnie Evangelista. I'm with the chief digital and artificial intelligence office, joined by Ramsey Brown. Sir, good, sir, can you introduce yourself, tell us what company you're with and what are you doing in this space?
Ramsey Brown [00:00:16]:
Yeah, thanks, Bonnie. My name is Ramsey Brown. I'm the chief executive officer of mission Control AI. We're a dual use generative AI operations prototyping, training and security platform for defense and commercial space. And we are here at the AI Advantage Expo to get to learn more from the Department of Defense and our community about how warfighter procurement operations staff are thinking about generative AI and what the heck to do with a language model. Yeah, that's what everyone's looking at right now.
Bonnie Evangelista [00:00:49]:
Yeah. So DoD Advantage 2024, I think it's our first one. There's a lot of content here going on. We were just talking off mic how it can be overwhelming sometimes. So how do you pick and choose your own journey or what you go after? What are you hoping to get out of this event?
Ramsey Brown [00:01:10]:
So, for us, we're a software technology company. We're up on the tradewind solutions marketplace. Our goals are around understanding the research and development needs, around testing and evaluation, and getting to find that out, not from a whiteboard or pure theory, but by talking to the teams and the units who are beginning to look at how they can integrate this technology into what they do and are running into roadblocks. And it's only by chatting with them and learning, okay, here's where we get stuck. Here's what needs to happen. Here's what we need a technology platform to do that we get that data that we can't just divine from the chicken bones or the wind. You have to go talk to people about that. And it's part of a startup's job, is to be able to directly interface with the teams that are going to be actually getting value out of what you build, meet them, understand what they need, be able to bring that back into the laboratory and build that out so you can deliver new capabilities that the DoD is looking for.
Ramsey Brown [00:02:07]:
But otherwise it is sitting around and saying, how do we make sense of this new tool and how do we do so in a way that maps to the requirements we're actually seeing.
Bonnie Evangelista [00:02:16]:
So you're talking about integrating cutting edge with people's existing day to day or giving them that. I've heard other people describe that ten x of improvement in their day to.
Ramsey Brown [00:02:28]:
Day life, and that's what we've come to find, probably unsurprisingly to anyone who's worked at any technical edge for very long is that while a lot of folks think that the hardest parts of all of this will permanently be that the technology capability is almost there, or the models do what they're supposed to, but not quite well enough, all of those technical problems and edge problems fall over pretty quick to R D. There's a very, very deep talent pool in the United States right now working on these technologies that are pushing forward their capabilities really fast. Perennially, what the problems end up being are around the. So what of all of this make this work for my daily life as an operator or a contracting officer or a mission planner or a strategist or an analyst, find ways that this actually is useful for me on my day to day basis, on my day to day life, that part is hard. And then for organizations that are looking at this and saying, how do we change and adapt once we've gotten an ato, once we've gotten some clarity to use this tech, how do we change and adapt to modernize and be able to pull these capabilities in, not to the technical level, but then build the capabilities bottoms up for making sure that we don't just have people who are now stepped up in, okay, great. Everyone knows a little more about data, but they have the new skills to get to use this entire new generation of technology. Those people problems tend to actually be what's inside all of these technology problems. That's where the rubber has to hit the road and people have to be able to say, yeah, now I get what I'm supposed to do with this thing.
Bonnie Evangelista [00:04:01]:
Can you give an example of some of those people problems? Because I would like to live in a world where if somebody was proposing to improve my daily life in some form or fashion, we would be all for that. But I think you're suggesting that's not what happens in practicality.
Ramsey Brown [00:04:16]:
And I don't think it's anyone's fault, per se. What I think is that a lot of people look at technologies from a top down perspective and say, oh, good, a large language model or a generative AI system, surely this can do something for me. And by starting at the top of that pyramid with what's the tech made of, and then try to work backwards to their daily life, something gets lost in translation. If you flip this model and say, here's my daily life, here's the job I operate, here's the handful of behaviors that go into what makes up my work week, and then you work forward to and tell me what a language model can do again, you will always find a pathway to being able to use a new tool. So what we see working is when people flip that model from, oh yeah, I bought the shiny thing. What do I do with the shiny thing? To, okay, what do I actually need to get done on a day to day basis? And then you work forwards to, okay, now I found an entry point for this shiny thing into what I already had to do to start with. So we see this happen across almost every job role, in the commercial sector, but also in the defense sector, around the digital workforce, where their jobs revolve around being able to ingest information, make good decisions, efficiently, execute on the day to days of the digital operations that make their unit move forward. All of those are jumping off points for a knowledge worker to be able to integrate a new tool like generative AI once they've got that specific clarity around that aha.
Ramsey Brown [00:05:48]:
Moment of, oh, here's what I was already doing to begin with. Now I see how this maps in versus handing them. Here's a new hammer, figure out what nails to hit with it.
Bonnie Evangelista [00:05:57]:
How are you thinking about some of the perceptions around llms in particular? So, especially as it gets introduced into the settings you're talking about, like practitioners, end users, soldiers, there's a lot of questions about security or responsible AI. How are you thinking about that in terms of you're in a startup setting and you're trying to get people to adopt this way of doing business.
Ramsey Brown [00:06:27]:
So the first way we think about this is from the sheer perspective of what is a large language model. We are of the opinion that these are fundamentally dual use technologies, and it's hard for us to talk about the civilian and commercial applications without also keeping in mind the immediate defense and security applications and advances that are getting made in the capability set of large language models in terms of their sophistication or their accuracy to us can be viewed through the lens of these are advances in weaponry and these are advances in systems that we need to have hard conversations and guardrails around as they continue to develop and then open source out into the community and then find their way into the hands of either civilians or to peer near peer adversaries. And imagining that world helps us understand what are the necessary steps we need to be taking today to secure that place we're stepping into. So that's the first things we think about these. These are intrinsically dual use. It's not an afterthought that is intrinsic to their behavior. From the perspective then of how do we secure this for day to day operations, especially within a defense context. We think about superficial trust and deep trust.
Ramsey Brown [00:07:44]:
Superficial trust, despite the term superficial, just meaning surface layer, is things like operational security. If I'm using an LLM, do I have it in an environment that has the proper security levels built around it to be able to use different types of information, whether that's il four, il five, where I know that this thing I can use as a language model can be used in a context that is approved for the data I'm putting into it, and that's a superficial form of trust. If I'm not using it in one of those contexts, am I able to secure it by filtering out information that might go into it or things that might come out of it that could cause some problems? Are we able to monitor for the hallucinations that the models create that are intrinsic to their performance, that right now and into 2024, 2025, there's probably no way around. We're just going to have to contend with hallucinations. Can we at least detect them, flag them, and provide that to human operators so they know? Okay, so far this answer was good, but here's where it tripped up. And now that I know this is where it can be detected to be tripping up, I know how to operate around that without losing the utility of the tool. That's superficial trust. Deep trust is what went into the creation of the model itself.
Ramsey Brown [00:08:59]:
If you know that a large language model provided by. And I'm just going to pick on OpenAI or Microsoft here for chat, GBT and GPT four, if you know that model was trained on the comment section of Reddit. And the comment section of Reddit has been demonstrated to be rife with dis and misinformation as detected by the open source intelligence community and is constantly the conversation point of the hybrid warfare community. And this model now contains those concepts trapped in language about potentially strategic concerns for which you have us defense operators relying on this model to answer questions about their day to day lives or strategies they should be taking or pathways they should be executing. And this model contains patently un american concepts or ideas. There's no amount of guardrails you put up against that thing to allow someone to deeply trust its behavior. You're going to be starting from scratch if you want to build something that can be truly, deeply validated, to say, yes, we know all of the thoughts in this thing's head. We know everything it's seen, we know everything that it learned, and we can verify that that information strategically aligns with the outcomes that we're looking for.
Ramsey Brown [00:10:17]:
And we're not worried about whether or not it's seen half a four chan. We are certain it hasn't. We trained it on the patent office and the Library of Congress or something. Great. Those forms of superficial and deep trust are, I think, what the field's playing out right now and trying to understand between these two, are we able to both look at options for building out that superficial trust for human operator and providing at a deep model level, a set of technologies that we can verify? Yes, these are approved for strategic uses versus what the commercial sector has to offer.
Bonnie Evangelista [00:10:49]:
How do you think about model monitoring? Dr. Martell, in his keynote, was very specific about that is a key component to LLMs in particular. And how does that play into what you're talking about? So you have it, and hopefully we're building the right constructs around it so that to increase adoption.
Ramsey Brown [00:11:14]:
And then what right it needs to get into the hands of the operators. And that model monitoring has to happen at the testing and evaluation stages. So when we're figuring out, is this model approved for use to begin with, can we baseline it, not just about its performance in terms of how fast does it run or what sort of complex sentences can it create, or how does it work on tasks? But could we even do things like conversationally verify its behavior against a known set of potentially situationally contentious concepts or topics, not to see that it shies away from them, but how it makes decisions that we'd consider virtuously aligned or ethically aligned, or aligned with the responsible AI recommendations or requirements that are being put out by the CDAO's responsible AI group. You do that at baselining, and then you need something that's more analogous to the DoD's continuous ato model in cybersecurity that would allow you to continuously monitor the performance of these models as they're being used in the wild. So we're going to want something that's closer to a heartbeat monitor living on every single one of these models as they're executing, incapable of continuously verifying that they're not just online and working, but that they're working in ways that we can prove to ourselves, to a certain confidence interval, aligns with the virtuous requirements we've otherwise set forth, because they have a propensity to drift in their performance. If you change their hardware, their mission context, if you embed them in a cyberphysical system, all of these introduce new variants, and being able to move to something like a continuous ato type framework for this way of thinking is the only way we're going to be able to meet what Dr. Martel has described as the next requirement around us, knowing, yeah, I can trust this thing.
Bonnie Evangelista [00:13:00]:
Well, will you do a thought experiment with me?
Ramsey Brown [00:13:04]:
Hit me. How did you know I love thought experiments?
Bonnie Evangelista [00:13:07]:
I don't know if this is a true thought experiment, but I'm curious if you would walk me through, from your vantage point, of course. What is the generalized perception of how to use tools like chat GBT? And we'll start there. And then what I'm walking toward is, how are we trying to change that, those actions and behaviors or whatever? And in terms of what do people think they can use the tool? And I'm picking on that one because that's the most common one that people have heard of.
Ramsey Brown [00:13:39]:
Right.
Bonnie Evangelista [00:13:39]:
Of course, there are other startups like yourself, that are using the same tech to meet specific use cases just like you described. But I would be remiss if I didn't put on the table. So this thing is out there. Heard it described as the toothpaste is out of the tube, right?
Ramsey Brown [00:13:58]:
Yeah, I'll say that the genie is out of the toothpaste tube.
Bonnie Evangelista [00:14:02]:
Yes. So what is, from your vantage point, again, kind of the generalization where people are, like, the most common use case, and it doesn't have to be work. It could be, like, for your personal life, like I go to Chachi BT to do to build my workout or whatever. What is that looking like for.
Ramsey Brown [00:14:23]:
Intrinsically technical people who are maybe data scientists or engineers who are looking at this through the lens of, here's a new layer in my technology stack. Where can I plug it in somewhere to take something that used to previously be a human decision or human behavior and remove the human from that piece. So they're looking at this as, here's my hammer. And this hammer hits invisible, hidden back end data tasks and just makes them go faster, or we have to do thousands of them an hour instead of doing them at human velocity. And the challenge with that is that these models are magic to the extent that they will continue to astound us as we come to understand exactly how big model latent space is and how many capabilities we haven't even found yet that they have even today that we're still uncovering, because we didn't design them to do any of these things. The source code of Chat GPT is something like 200 lines of code. It is a not sophisticated piece of core technology, but rather its sophistication is emergent of having read the entire Internet and figured out the latent statistical structure of human thought in a way that is functionally indistinguishable from synthesizing human thought. That part, if you ever look at old timey maps from the early days of global exploration, and at the edges of the maps, they would just draw dragons and sea monsters because they didn't know it was there.
Ramsey Brown [00:16:02]:
So it was a here be dragons. We're still pushing back the map of the known with what's going on in language models.
Bonnie Evangelista [00:16:09]:
Yeah.
Ramsey Brown [00:16:09]:
And even though that's true, from the data engineer's perspective, this magic tool surely could fix X, Y or z. And the reality is, it often doesn't, because they run into the same traditional data science or data engineering problems, that their pipelines aren't up to snuff for the task. Their data is in a legacy format or it's not usable. So these things don't end up being helpful because they're not a fix all. So that would be the first kind of common failure mode. The second is for people who do not think of themselves as having an AI job, is that they look at these things and say, but my job doesn't use AI. And that's the wild part, because even though you or I or a listener of defense mavericks has probably played around with or actively incorporates synthetic intelligence into the day to day of their job, or even the day to day of their lives, that is an anomaly for most people. Most people have heard of this technology and have not touched it right.
Ramsey Brown [00:17:09]:
And that is even now going on a year after GPT four's release and more than a year after Chat GPT, these are not really highly adopted models. Everyone's kind of loosely aware of it in the cultural background, or they hear Jimmy Kimmel crack a joke about it, but that's really different than I actually use it. So the stumbling block is that most people don't think of themselves as having a job that AI could touch. And if you use a keyboard for a living, if you have opened Microsoft Teams in the past 90 days, or slack or your email, or fill in the blank for whatever causes you stress, AI impacts your job, and not in a nebulous, the economists say ten years from now, something, something structural unemployment. But that there are specific, actionable ways where today's technologies are going to drive value for you as a digital worker to help you do more effective, faster work with less effort. And a lot of what we've come to understand at Mission control AI, is that if you give people a technology to uncover, oh, here's the ways that this does plug into the jobs that me or my unit or my team have to perform that provides the missing clarity or library of use cases that people were looking for around. Oh, so now I get what to do with this, because if you show it to them ahead of time and say, does this apply to your work? Say, well, no, I don't have to use that. As opposed to, yeah, but I could imagine the thousand ways this was going to make me better at my job or be able to do my job with less sweat.
Bonnie Evangelista [00:18:42]:
Okay, here's another one. Hit me. What would you say to somebody who says AI is going to make us stupider? I'll be specific. I was presenting to a group about contracting and how we were using certain tools that did integrate some AI type technology on the back end to do exactly what you're describing. And they said, but if we do that, then the workforce is just going to get stupider because we're not going to know why a happens to be or why certain clauses go into contracts or whatever. What do you say to that person?
Ramsey Brown [00:19:21]:
They may be correct, and that's a contrarian answer, because I work in AI and I'm a large proponent of accelerating AI adoption and capabilities. And so my job is to say, that's preposterous, certainly couldn't, but no, that's a very real possibility. And a man named Nicholas Carr wrote a book, and I want to say, about the 2013 2015 era called the Shallows, which proposed that search engines were changing the way we think. By having the world's information well indexed and organized and available at your fingertips, workers were becoming more effective at using Google at the cost of being less effective at thinking really deeply about problems. Mr. Carr proposes a really interesting hypothesis. It's unclear where the data actually mets out about do these tools make us stupider per se? Or if I used to have to spend a lot of hours per week being good at navigating a contract, and now there's a tool that will do an 80% job in one 100th the time, which allows me to get through a lot more work with a lot less effort. And my job moves from principal practitioner who had to push that forward, or something like supervisor of cyborg that has to check the work being done that is a different skill set getting exercised.
Ramsey Brown [00:20:52]:
And if that's what you spend most of your work week doing, that becomes an opportunity cost formula about the skills you could have been practicing that made you good at authoring that contract or reviewing that contract. And the brain operates in a use it or lose it kind of pathway. And the things we practice are the things we will get good at. Does generative AI make people stupider? No, but it will change things we practice. And if we substantially change the things we practice, we should expect to fall out of practices pretty fast, because that's how behavior works, and that's something we have to contend with.
Bonnie Evangelista [00:21:25]:
That's fair. So are you a proponent for more of this knowing and understanding? That's the trade off. We're going to have less practice skills in one area, but we'll have more practice skills in other areas.
Ramsey Brown [00:21:39]:
Yes. And if we look the five to ten years down the timeline, we see the capabilities curves on these models getting good enough, fast enough, in ways that would today appear to be functionally impossible. But we will come to find, like every other phase of AI's evolution, the models were relatively bad at something for a long time, and then kind of good at it for a little while, and then basically beyond human mastery, very fast. It's our professional opinion that for most human knowledge work tasks, our timelines of that are about five to ten years. And that means we have to contend with how to plan for that world. Is that a dig your heels in and start smashing looms and breaking data centers kind of mentality? No, that's never going to work. There's no incentive there that says that that's going to be a pathway that's even an option on the table. So instead, the question becomes, how do we look around some of these corners and say, at the five to ten year time span, what are the skills that people should be developing around these new technologies? Given that a lot of the things they've been doing for their careers, there's now going to be no demand for the human performance of those tasks.
Bonnie Evangelista [00:22:51]:
Right.
Ramsey Brown [00:22:51]:
We can look beyond the ten year horizon and say, we've got a serious question, if not problem, when language models are capable of doing most, if not all, human knowledge work tasks better than us until then, which is still a. If you think that 2023 was long, just imagine how long it's going to feel until 2033 or 2034. That's going to feel like 30 or 40 years of progress get done by then. And in that time, the jobs of the future we're going to find are going to happen really quickly, and most people are going to find themselves upskilling into more and more subtle ways of operating like a human machine hybrid team with their tools, as opposed to the relationship they might have previously have of just sending commands and then computer does thing, it's going to feel more and more like a colleague or like another knowledge worker working alongside you than it is like the relationship you might have with your microwave or your dishwasher. And as people build those skills and capabilities towards there, and as organizations turn towards that, they're going to find themselves ahead of that transformation and really well prepared.
Bonnie Evangelista [00:23:52]:
Man, are there any other common comments you get when you're interacting with more of that population that might be aware of stuff like this, but they're not using it, but they always have an opinion on it. Right. So what's, I don't know, top two or your favorite kind of narrative or comment you hear from people like that outside of.
Ramsey Brown [00:24:14]:
Yeah, but I don't use AI in my job. But my job doesn't need AI. That's not how that works. Your job can be made more productive through the use of these tools, and our team in our software can help with that. So there's that, but then there's the. Yeah, but I've seen AI and it's bad at X and fill in the blank. X is anything. Yeah, but it's kind of bad at writing limericks as of 2019, right? Or.
Ramsey Brown [00:24:36]:
Yeah, as of three days ago. Yeah, I know it can generate 30 seconds of 4k footage that's never, ever happened before. Once, just from a prompt. But don't ants have six legs and not four?
Bonnie Evangelista [00:24:47]:
Well, that's kind of one of my favorites, is if they do use the tool, they're like, oh, but this is wrong.
Ramsey Brown [00:24:54]:
Got you.
Bonnie Evangelista [00:24:54]:
I stumped it.
Ramsey Brown [00:24:55]:
Yeah, because, well, there's something. I'm going to be completely real with you. I think it's like a fear response thing from an apex predator looking at something coming for its turf. Because for the first time, we're contending with a thing like, if you showed the naysayers of AI the capabilities today and asked them, what year did that happen in? What year was this commercially available such that idiots on Twitter were writing about it, their answers are going to be 2092 100. Everyone's goalposts are moving really fast. And so when someone says, yeah, but it can't possibly do x, but look at how terribly it does x. Remind them that one year ago, the current state of the art in something like video generation looks like nightmare fuel. It was just hilariously terrifying how bad the models were, and now they're becoming functionally indistinguishable from reality.
Ramsey Brown [00:25:46]:
If we remember how bad GPT-2 was and how almost useless these tools are not a decade or three decades later, but like four years later. Now we have tools that are good enough that we're having to throw away the Turing test because it's not helpful anymore. So when people say, yeah, but it can't do x, it's like you need to give it six months. And the fact that I'm asking you to give it six months, not 60 years, should be telling to us that this is the world we operate in and that's where we need to build preparedness for.
Bonnie Evangelista [00:26:12]:
Yeah, I think it's really interesting how it's happening so fast. Like you said, wasn't Chachi BT's birthday? Was it back in November?
Ramsey Brown [00:26:23]:
Yeah, that was one year. It will be March 15 or 16th since GPT four coming out. And everyone loves saying, oh, exponential change or accelerating change. And I think what they narrowly mean is this post Ted Talkian Steve Jobs 2011 2012 time loop about something something VR goggles, something something drones, something something tablet computer, and we anchor on gadgets. That's what we meant when we said like the future capital f, as opposed to wow. Every day that passes will contain slightly compoundingly more change in innovation than the day before it. And like every other thing that compounds, what that means is that especially as non human synthetic intelligence systems start being able to be principal actors and autonomous actors in that world, we're going to have billions of semiautonomous minds, and not in the 2040 timescale, but in the 2025 2026 timescale, capable of at non human speeds, capabilities, depths and accuracies and breadths, pushing forward fundamental research and development in almost every facet of the human endeavor. And if we think that this feels fast today, we haven't seen anything yet, because this is all still being pushed forward by people, and it still feels like it's speeding up.
Ramsey Brown [00:27:46]:
To really embrace that we live in a time defined by accelerating change means that every day, for the rest of your eyes, is going to feel faster than today, and each day is going to feel faster than the last. That's what accelerating means. That's a hard thing to contend with.
Bonnie Evangelista [00:28:01]:
It is. I also wonder for some of those people who maybe just for whatever reason, are not as involved or interested or think it applies to them, if they're aware of how much it's still around them. Lots of companies and more of maybe their personal life are using these things, and it is affecting them, whether they know or realize it or not.
Ramsey Brown [00:28:27]:
I feel like a lot of people do, in the back of their minds, know these things to be true. I think outside of the technical literacy or use case job literacy, like what we're describing here, what we work on there is that greater cultural literacy and feeling of a low sigh of control internally that they're still kind of in control of their life up against this, and everyone's losing that very quick for.
Bonnie Evangelista [00:28:53]:
People maybe who are not grasping what I'm talking about. I always go back to contracts, that's what I know. And I tell people all the time, if you think people in industry are not using these tools to write proposals, you're in denial or you're just to your point, not choosing not to be aware of this. And I love the ones that maybe are aware of it, and you make great point about trying to control it. They try and put in the solicitations like we will not accept proposals that can you enforce. Thank you.
Ramsey Brown [00:29:23]:
What's funny is now we're starting to read papers on archive from colleagues or other companies, and you read them and they smell like generative AI, like they have this language style, a little stink to it, a little stanky, but they have this way of speaking that is very clear that no human copy editor went through after the fact, and at least they didn't include the well as a large language model developed by OpenAI. Part of the problem. But when people dig their heels in, I think that's a natural thing to want to do. And we are relatively vocal that we think this change is not an incremental, like, oh, we went from like dial up to cable kind of thing, or slightly shit wifi to slightly less shit wifi. We think this is actually, and we have good reason to believe that imbuing physical inanimate matter with the spirit, creativity, purpose and mental competency of human beings, whether an economically usable or militarily useful simulacra or emulation or the real thing, is a philosopher's conversation, and we're not having it, but it's useful and people are buying it, and it's good and practical. That's likely one of the most important things human civilization has ever done on par with the agricultural revolution or the industrial revolution and the electrification of the world. This is one of those singular types of moments for which the amount of change this unlocks on the human condition, on the biosphere, and on a relationship between the two is going to be in those few things a civilization gets to do right exactly once. And it's why, as a team working on securing AI and AI governance, we take our work as seriously as we do.
Ramsey Brown [00:31:09]:
So when we look at things like task Force Lima, or we look at things like the replicator initiative, and we look at the work that CDAO is putting out around responsible AI, we see these as being non optional parts of doing everything within our control to make sure that this technology remains well and virtuously aligned to the things that make America and its allies strong and respect the dignity and positive and negative freedoms intrinsic to our lives, as opposed to, well, let's just see what the market says, let's just see what the technology takes us. Let's just let it rip. That's a non tenable solution. If you're embedding autonomous systems in cyberphysical systems or kinetic platforms, there's no let the market find out kind of version of that reality. And as I said in the beginning, if we really believe that these language models are dual use systems, we need to be embedding that level of conscientiousness and thoughtfulness into every stage of their use. Whether that's something extremely mundane or something very sophisticated. We view that as being table stakes for how important this thing is that's going on. And it would be understandable for someone having one of those few times in a civilization's history level events to look at it and quietly say, yeah, I'm just going to pretend this isn't happening.
Ramsey Brown [00:32:18]:
I'm just going to pretend that I get to live through the 1990s, part two. Or wouldn't it be great if I kind of lived a life that was like my parents lives or my grandparents lives, as opposed to everything around me is changing in real time, and I feel like I'm grasping for straws for how to maintain a semblance of understanding, identity, peace and control in that world. I get why people want to put the head in the sand, want to put on the sunglasses, to block it out, because at times it can be like staring at the sun. But it's also our responsibility to do that. If we have this singular moment to be those better versions of ourselves and make this thing productive and virtuous, that means we've got to stare at the problem and we've got to embrace it, and we've got to figure out what to do to make sense out of this thing and make sure that we are safer and more secure with it.
Bonnie Evangelista [00:33:00]:
Solid perspective. I want to do a little bit of a 180, though, and then I want to close on maybe a little happier note. It's been very heavy, right? With all the AI and the tech. One of the last conversations we had, you had some solid tips on longevity. What are your top three things we could be doing to live a longer, better life? And just so everyone knows, these are not arbitrary, you could talk a little bit about where you got these from.
Ramsey Brown [00:33:27]:
Yeah, there's a really wonderful way to round this out. Thank you. I love that. I was not expecting. Yeah. For context, I was working on a computational neuroscience PhD at the University of Southern California. And because I was a broke ass grad student, I ended up a teaching assistant quite often. And I was a teaching assistant in a neuroscience of gerontology course.
Ramsey Brown [00:33:47]:
And gerontology is the study of aging. So how do we age? And what does it mean for a brain to age? And half of our students would come in expecting that we were going to tell them the secret to living forever.
Bonnie Evangelista [00:33:59]:
And this is biohacking.
Ramsey Brown [00:34:00]:
This is like pre Dave Asprey, pre Brian Johnson, pre blue blockers and keto. But we would always go back to some basics that were put forth by the author Michael Pollan and some of his really wonderful books about our relationships to food, because that's a really great place to start. And we built upon that for some really helpful insights from the behavioral sciences and population health studies. His advice is really straightforward, and that's the best part of all that, because it's like the least AI thing humanly possible, right? Number one, eat real food. Twinkies and Big Macs don't count. Sorry. I know they're delicious. Not too much.
Ramsey Brown [00:34:45]:
Mostly plants. Not all plants. Just mostly plants.
Bonnie Evangelista [00:34:49]:
Mostly plants.
Ramsey Brown [00:34:50]:
And then everything that wasn't about food crashed out of the literature was the stuff that our grandparents were good at. Have friends. Spend time in the sunshine. Get adequate sleep that does not involve falling asleep to the office on your iPad. Move your body pretty routinely. Be able to be strong at every age. Do not treat your physical strength or your ability to do resistance strength as something that is either masculine coded or you're not training for anything, so you don't need it. Be able to put up good resistance.
Ramsey Brown [00:35:24]:
Make sure your friends also know one another, such that you don't have a hub and spoke model of relationships in which you're the center of a lot of folks, but rather you have a redundant network such that people will do things like check up on. Hey, have you heard from Bonnie in a few days? I've heard from her. Turns out those things really well. Predict things like the recovery from postpartum depression. Be involved in a faith community as one's super uncomfortable for millennials and anyone younger than millennials, because I would love so much baggage. So much baggage. God, that was for my dad. But find something bigger than yourself to believe in, because it turns out it's not that divine intervention is going to prevent your cells from falling apart, but rather the peace and support of community lowers cortisol levels.
Ramsey Brown [00:36:11]:
Right. Drink, but not too much. So the old studies around, oh, red wine, because of the tannins, something, something. Raboflavin, something antioxidants. Turns out that if you control for all of those things and all cause mortality, people who just drank two drinks a night of anything just dramatically outlived everybody else. That's not actually medical advice because of.
Bonnie Evangelista [00:36:32]:
Stress, they're just relaxing.
Ramsey Brown [00:36:33]:
According to these studies, this is about the stress reduction as opposed to something magical from Italy. So you're having your two gin and tonics a day. That's great. Be close to people. Find ways to build emotional and physical intimacy in your life. Do all the things that basically every other generation before us has managed to get their head around. There's not going to be some injectable, there's not going to be some genetic treatment, there's not going to be some miracle. No silver that you fall.
Ramsey Brown [00:36:58]:
There's no silver bullets, there's no bulletproof anything. It's the stuff that just. We already have an intuition for. Makes us well. It's like, I really wanted there to be something secret. No, there's no secret.
Bonnie Evangelista [00:37:08]:
No.
Ramsey Brown [00:37:09]:
That's the best part of it.
Bonnie Evangelista [00:37:10]:
I love what you said about strength because there is some research out there that says your ability to get yourself off the floor is actually an indication of.
Ramsey Brown [00:37:22]:
I'm so glad, you know this. This is one of my favorite things in the world. And when I go to strength training and we have to do any floor mat work, this is the only way I get up for listeners. This is something that has been found to be a really helpful predictor of where someone is in degeneration when they're really elderly, is if they sit down on the floor of a doctor's office, can they get themselves up without using.
Bonnie Evangelista [00:37:41]:
Their hands or a table?
Ramsey Brown [00:37:43]:
Or a table?
Bonnie Evangelista [00:37:44]:
Yeah, that's your hands.
Ramsey Brown [00:37:45]:
All you have to do is just not use your hands or your arms to get up. So if you are capable, at any age, of getting your body off the ground without your hands, that, it turns out, is really helpful. Health predictor that, like, okay, it might have other problems, but it's basically still ticking, these little things.
Bonnie Evangelista [00:38:02]:
I appreciate you sharing that. I know that we kind of, like I said, did a 180 but that.
Ramsey Brown [00:38:06]:
Was about as 180 as we could have gotten. But it was a lot of fun, too.
Bonnie Evangelista [00:38:08]:
I think it's the perfect place to end.
Ramsey Brown [00:38:10]:
Yeah. Bonnie, thanks so much for having me. This has been a ton of fun. All right.